I wanted to make this post for a while, as I am deeply invested in the development of AI image models, but things happened so fast.
It all started in January 2021 when OpenAi presented DALL-E, an AI model that was able to generate images based on a text prompt. It did not get a lot of attention from the general public at the time because the pictures weren’t that impressive. One year later, in April 2022, they followed up with DALL-E 2, a big step in resolution, quality, and coherence. But since nobody was able to use it themself the public did not talk about it a lot. Just one month later google presented its own model Imagen, which was another step forward and was even able to generate consistent text in images.
It was stunning for people interested in the field, but it was just research. Three months later DALL-E 2 opened its Beta. A lot of news sites started to write articles about it since they were now able to experience it for themself. But before it could become a bigger thing Stability.Ai released the open-source model “stable diffusion” to the general public. Instead of a few thousand people in the DALL-E beta, everybody was able to generate images now. This was just over a month ago. Since then many people took stable diffusion and built GUIs for it, trained their own models for specific use cases, and contributed in every way possible. AI was even used to win an art contest.
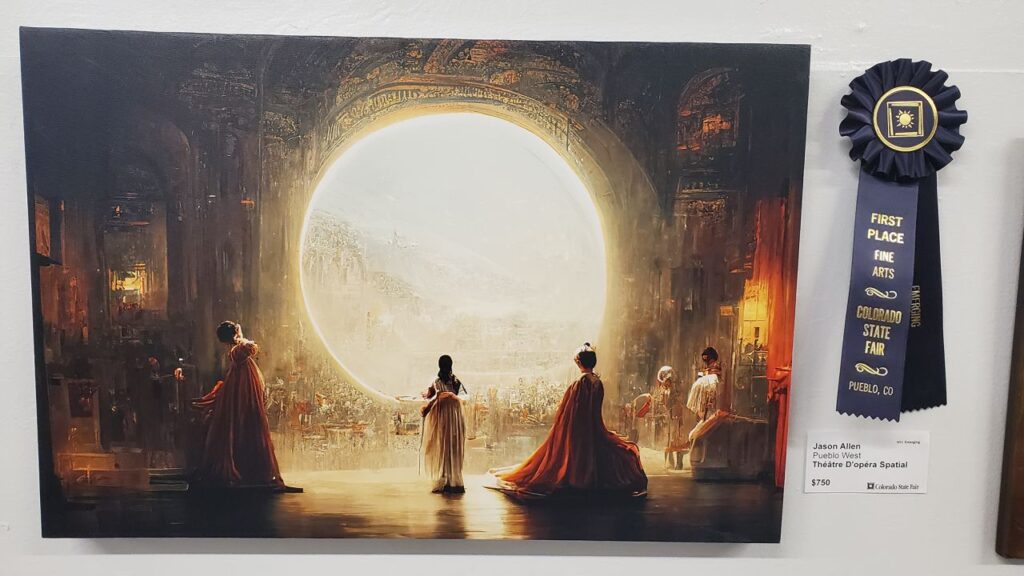
People all around the globe were stunned by the technology. While many debated the pros and contras and enjoyed making art,
many started to wonder about what would come next. After all, stable diffusion and DALL-E 2 had some weak points.
The resolution was still limited, and faces, hands, and texts were still a problem.
Stability.ai released stable diffusion 1.5 in the same month as an improvement for faces and hands.
Many people thought that we might solve image generation later next year and audio generation would be next.
Maybe we would be able to generate Videos in some form in the next decade. One Week. It took one week until Meta released Make-a-video, on the 29th of September. The videos were just a few seconds long, low resolution, and low quality. But everybody who followed the development of image generation could see that it would follow the same path and that it would become better over the next few months.
2 hours. 2 hours later Phenki was presented, which was able to generate minute-long videos based on longer descriptions of entire scenes.
Just yesterday google presented Imagen video, which could generate higher-resolution videos. Stablilty.ai also announced that they will
release an open-source text2video model, which will most likely have the same impact as stable diffusion did.
The next model has likely already been released when you read this. It is hard to keep up these days.
I want to address some concerns regarding AI image generation since I saw a lot of fear and hate directed at people who develop this technology,
the people who use it, and the technology itself. It is not true that the models just throw together what artists did in the past. While it is true that art was used to train these models, that does not mean that they just copy. The way it works is by looking at multiple images of the same subject to abstract what the subject is about, and to remember the core idea. This is why the model is only 4 Gbyte in size. Many people argue that it copies watermarks and signatures. This is not happening because the AI copies, but because it thinks it is part of the requested subject. If every dog you ever saw in your life had a red collar, you would draw a dog with a red collar. Not because you are copying another dog picture, but because you think it is part of the dog. It is impossible for the AI to remember other pictures. I saw too many people spreading this false information to discredit AI art.
The next argument I see a lot is that AI art is soulless and requires no effort and therefore is worthless. I, myself am not an artist, but I consider myself an art enjoyer. It does not matter to me how much time it took to make something as long as I enjoy it. Saying something is better or worse because of the way it was made sounds strange to me. Many people simply use these models to generate pictures, but there is a group of already talented digital artists who use these models to speed up their creative process. They use them in many creative ways using inpainting and combining them with other digital tools to produce even greater art. Calling all of these artists fakes and dismissing their art as not “real” is something that upsets me.
The last argument is copyright. I will ignore the copyright implications for the output since my last point made that quite clear. The more difficult discussion is about the training input. While I think that companies should be allowed to use every available data to train their models, I can see that some people think differently. Right now it is allowed, but I expect that some countries will adopt some laws to address this technology. For anybody interested in AI art, I recommend lexica.art if you want to see some examples and if you want to generate your own https://beta.dreamstudio.ai/dream is a good starting point. I used them myself to generate my last few images for this blog.
Text2Image/video is a field that developed incredibly fast in the last few months. We will see these developments in more and more areas the more we approach
the singularity. There are some fields that I ignored in this post that go in the same direction that are making similar leaps.
For example Audiogeneration and 2D to 3D. The entire machine learning research is growing exponentially.
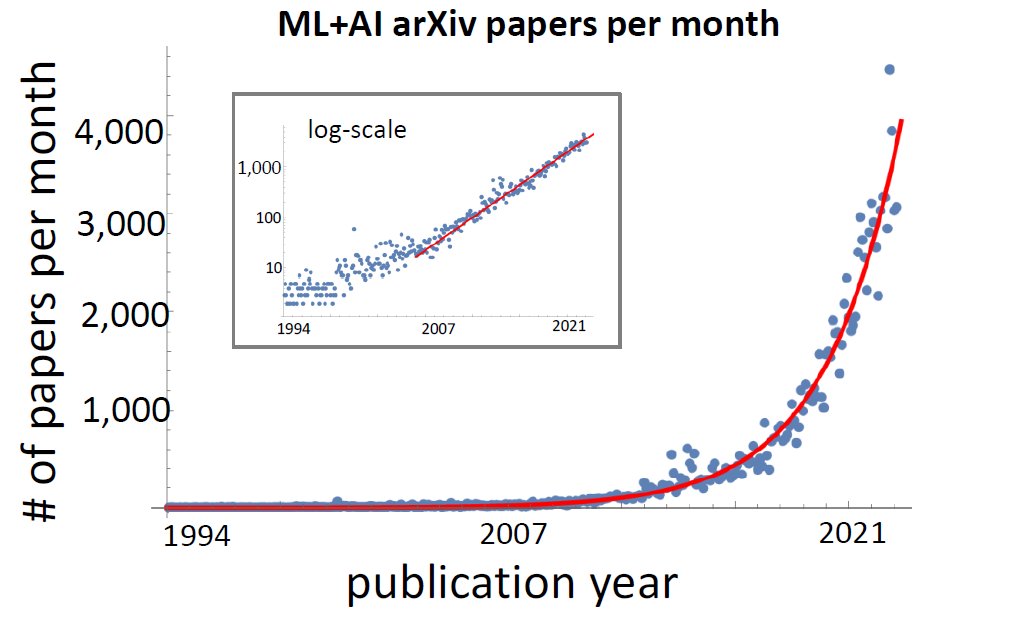
The next big thing will be language models. I missed the chance to talk about Google’s “sentient” AI when it was big in the news,
but I am sure with the release of GPT-4 in the next few months, the topic will become even more present in public discussions.
Leave a Reply