As we close the chapter on 2023, it’s time to revisit the predictions I laid out at the beginning of the year. It was a year marked by technological strides and societal challenges. Let’s evaluate how my forecasts stood against the unfolding of 2023.
Let’s start with my predictions about AI:
“AI will continue to disrupt various industries such as search and creative writing and spark public debate about its impact, even more than is happening right now. It will also lead to the production of high-quality media with fewer people and resources thanks to AI’s assistance. In the field of 3D generation, I expect to see similar progress in 2023, bringing us closer to the quality of 2D generation.“
I think I was mostly right. GPT-4 definitely sparked a public debate and we see many industries that became more productive thanks to AI. 3D generation is also already at the level that image generation had at the beginning of the year. What I did not predict was the speed at which companies like Meta or Microsoft would iterate and deploy LLMs in many forms.
My next prediction was about Fusion: “While I expect to see continued progress in this field, it is unlikely that we will see a commercial fusion reactor within the next two years.“
Again I was on point but I missed talking about other energy sources like solar which are more relevant. I would count that as a bad focus and not a failed prediction.
I also made predictions for Hardware: “[…] we can expect to see quantum computers with over 1000 Qbits in the upcoming year. GPUs will become more important with the rise of AI. However, these advancements in hardware technology also come with the need for careful consideration and planning in terms of production and distribution. “
We indeed achieved 1000 Qbits even though IBM was not the first company to do so. I also correctly predicted the increased demand for GPUs, but I have to admit I did not expect that scale. I also was more pessimistic about the ability of TSMC and others to meet the demand, and while they drastically outperformed my expectations I was still kind of right because the demand is also way bigger than I anticipated.
My Predictions for VR: “But the year 2023 is shaping up to be a promising one for the VR hardware market, with multiple new headsets, such as the Quest 3, and maybe even an Apple Headset, set to be released. These new products will likely offer improved graphics, more intuitive controls, and a wider range of content and experiences. While it may not fully realize the vision of a “Metaverse”, VR is still likely to be a great entertainment product for many people”
And AR: “2023 will be a critical year for AR. It will be the first time that we can build affordable Hardware in a small form factor. Chips like the Snapdragon AR2 Gen 1 implement Wifi 7 and low energy usage and will make it possible to build Smart glasses.“
While my VR predictions were all correct, my AR predictions underestimated the difficulty of producing smart glasses in a normal form factor.
I did not make concrete predictions about Brain-computer interfaces, but I honestly expected more progress. More about that in my new predictions later.
Now on to biology and medicine. I made a multiple-year prediction: “If this continues we will be able to beat cancer in the next few years, which leads to the next field.” this cannot be verified yet, but I still believe in it and predicted that a person under 60 could live forever. Recently I looked a lot more into aging research and I still believe that this is correct even though I would change from “every person under 60 has the potential“, to “there is a person under 60 that will“. I think this is an important distinction because stopping aging requires a lot of money and dedication and will not be available for most in the near future.
I ended the post with: “While this was a slow year in some aspects, major progress was made in most fields, and 2023 will be even faster. We are at the knee of an exponential blowup and we are not ready for what is coming. While I am still worried about how society will react and adapt, I am excited for 2023 and the rest of the decade.“
Again I believe that I was very much on point with this. Many people were blown away by the rapid developments this year. So let’s talk about the stuff that I did not predict or ignored last year. LK99 is a material that was supposed to be a room-temperature superconductor. At the current time, this was most likely false, but I realized that I did not make a prediction about superconductors in the blog post. I will do this later in this one.
On to the new predictions for 2024. Let’s start with AI again. LLM-based systems will become more autonomous and will reach a point where many will consider them AGI. I personally do not think that we will reach AGI this year, but most likely in 2025. There is also a 70% chance that we will find a new architecture that generalizes better than transformers. No system in 2024 will outperform Humans on the new GAIA benchmark, but they are going to double their performance on it. This will mostly be accomplished by improving reasoning, planning, and tool use with improved fine-tuning and new training strategies.
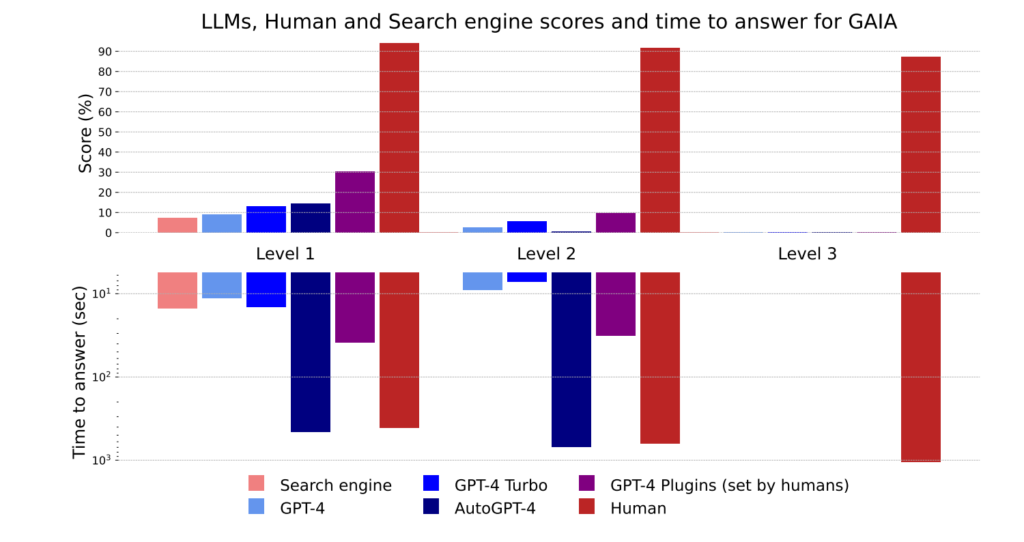
I also predict that commercially viable models will stay under 1 trillion parameters in 2024. There will be a few models over this threshold, but they will not be used in consumer products without paying for them similar to GPT-4 (non-turbo). State space models like RWKV will also become more relevant for specific use cases and most models will at least support image input if not more modalities. RL Models like Alphafold will push scientific discovery even faster in 2024.
Image/video/music/3D generative models will improve dramatically and completely change the art industries. The focus is going to be more on integration and ways to use them and less on pure text2output capabilities. Assistants like Alexa will integrate LMMs and improve drastically. OpenAI will release at least one model that will not be called GPT-5 and wait with GPT-5 until later in the year.
Apple will announce its first LMM at WWDC and at the end of the year we will be able to do most stuff by just talking to our PC. Meta will release Llama-3 which is going to be multimodal and close to GPT-4, and Google will release Gemini at the beginning of the year, which will be comparable to GPT-4 at the beginning and will improve down the year.
Open-source models will stay a few months behind closed-source models, and even further in areas like integration, but offer more customizability. Custom AI hardware like the AI Pin will not become widespread, but smartphones will adapt to AI by including more sensors and I/O options, and towards 2025 we will see smart glasses with AI integration. The sectors that will be influenced the most by AI are education and healthcare, but in the short term, the first industries will be artists and some office workers.
Let’s continue with Hardware. Nvidia will stay the leader in AI hardware with H200 and later this year with B100. Many companies will use their custom chips like Microsoft, Apple, and Google, but the demand will lead to increased sales for every chip company. At the end of 2024, more than half of the global flops will be used for AI. VR Hardware will continue to improve, and we will finally see the first useful everyday AR glasses towards the end of 2024. Quantum computers will become part of some of the cloud providers and will be offered as specialized hardware just like GPUs (Note: This part was written before the AWS Event announcement). They will become more relevant for many industries as the number of Qbits grows. We will also see more variety in chips as they become more specialized to save energy. Brain-computer interfaces will finally be used in humans for actual medical applications.
I did not make any predictions about robots last year, because there weren’t many exciting developments, but that changed. Multiple companies started developing humanoid robots that will be ready in 2024 or 2025. I expect an initial hype around them and adoption in some areas. However, towards the end of the decade they will be replaced with special-purpose robots and humanoid robots will be limited to areas where a human form factor is needed. In general, the amount of Robots will increase in all areas. Progress in planning and advanced AI allows for robots to act in unknown environments and do new tasks. They will leave controlled environments like factories and will appear in, shops, restaurants, streets, and many other places.
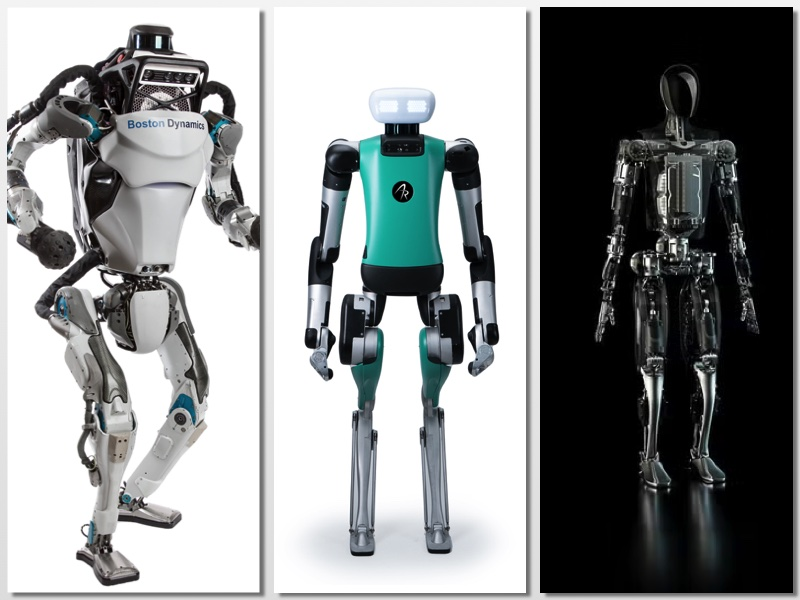
Let’s continue with energy. The transition to renewable energy will accelerate in 2024, with a significant focus on solar. The first commercial fusion reactor will begin construction, and nuclear reactors will become even safer, mostly solving the waste problem. More people will build solar for their own houses and become most self-sufficient.
I mentioned LK99 earlier already so here are my predictions for material science. I think that if a room-temperature superconductor is possible, an AI-based system will find it in the next two years. In fact, most new materials will be hypothesized and analyzed by AI and will bring a lot of progress for areas like batteries, solar panels, and other material-dependent fields (Note: this part was written four days before Deepmind presented GNoME).
Biology and medicine are poised to make significant leaps, powered by AI systems like Alphafold and similar technologies. Cancer and other deadly diseases will become increasingly treatable and aging will become a target for many in the field. The public opinion that aging is natural and cannot/should not be stopped will not change this year but maybe in 2025. Prostheses will become more practical and will be connected directly to nerves and bones. This will make them in some areas better than human parts, but touch and precision will continue to be way worse. We will also see progress in artificial organs grown in animals or completely made in a lab.
Transportation in 2024 will change slightly. EVs will become more popular and cheaper but will not reach the level of adaptation that they have in China. Self-driving cars will stay in big cities as taxi replacements and will not be generally available until 2025. Hypertubes will not become a train replacement and will only be built for very specific connections if they get built at all in the next few years.
Other infrastructures like the Internet will continue to stay behind the demand for the next few years. The main driver of the increased need for bandwidth will be high-quality video streaming while the main need for speed will arise from interactive systems like cloud-based AI assistants.
Climate change and unstable governments will lead to an increase in refugees worldwide and social unrest will increase. We will see the first effects of AI-induced Job losses. The political debate will become more heated and some important elections like the US election will be fully determined by large-scale AI-based operations that use Fake news, Deepfakes, and online bots to control the public opinion.
I made a lot more verifiable predictions this time and I hope to see how much I got correct. If I missed any area or technology write them in the comments and I will add a prediction in the comments. Also, let me know your predictions.